Objective
The popularity of task-oriented chatbots is constantly growing, but smooth conversational progress with them remains profoundly challenging. In recent years, researchers have argued that chatbot systems should include guidance for users on how to converse with them. Nevertheless, empirical evidence about what to place in such guidance, and when to deliver it, has been lacking.
Read Paper➝
Outcome
- It establishes that each guidance type and timing has particular strengths and weaknesses, thus that each type/timing combination has a unique impact on performance metrics, learning outcomes, and user experience.
- On that basis, it presents guidance-design recommendations for future task-oriented chatbots.
Deliverables
- As one of a UX Researcher in this team, I cooperate with 5 UX researchers, and I in charge with qualitative part. We use affinity diagram to analysis the qualitative data and generate insight integrated with quantitative data.
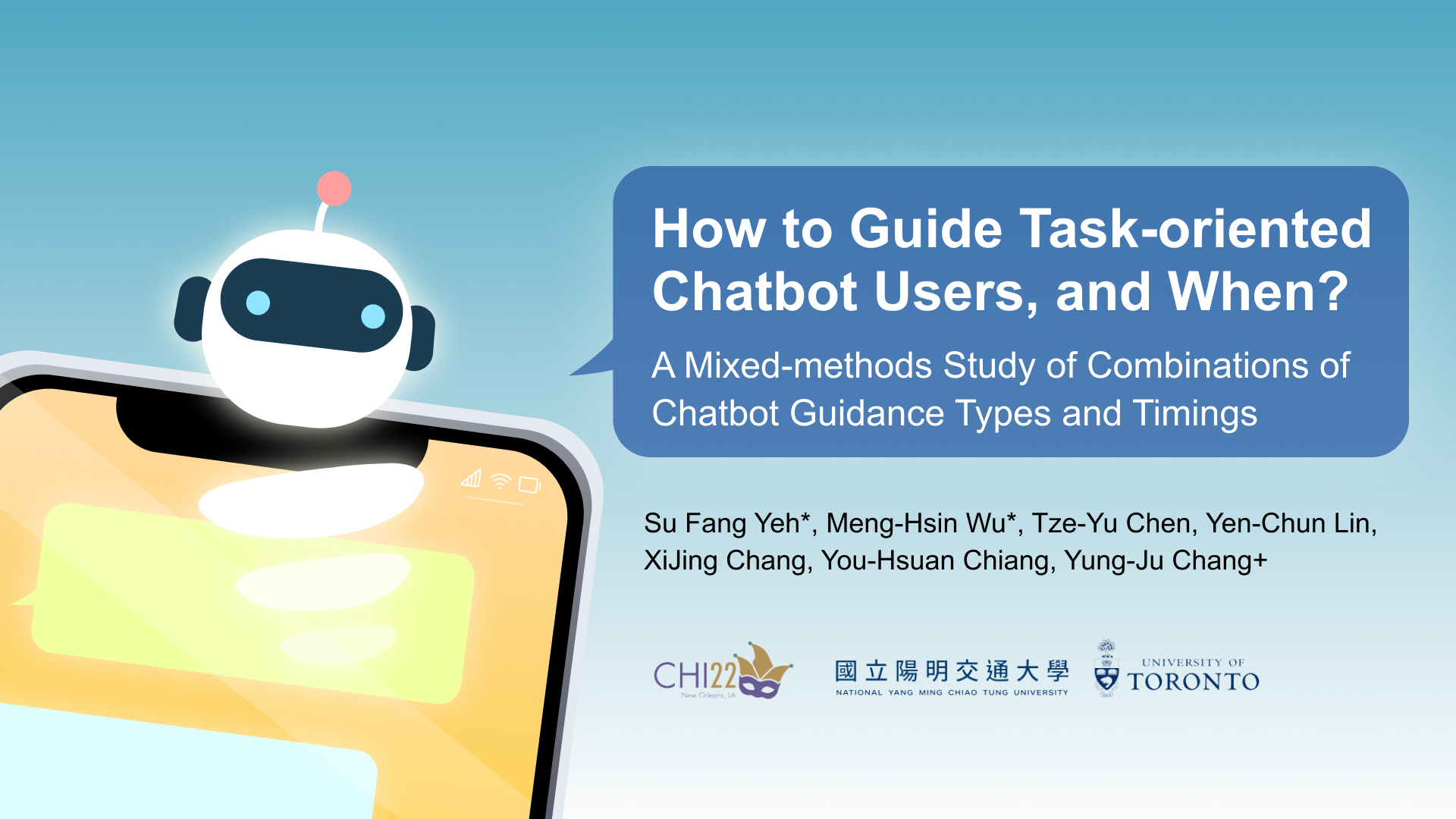